WWW.YOUJJ,CON
WWW.YOUJJ,CON
Templates
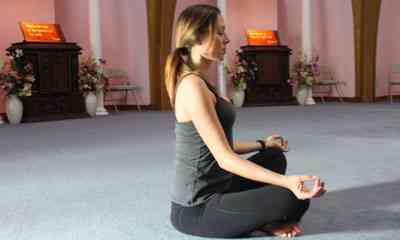
Popular Meditation
Lorem ipsum dolor sit amet consectetur adiing elit. In volutpat luctus eros ac placerat. Quisque erat metus facilisis non feu,aliquam hendrerit quam. Donec ut lectus vel dolor adipiscing tincnt.
More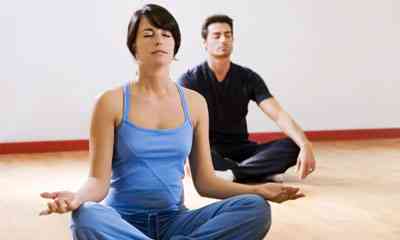
Stillness yoga
Lorem ipsum dolor sit amet consectetur adiing elit. In volutpat luctus eros ac placerat. Quisque erat metus facilisis non feu,aliquam hendrerit quam. Donec ut lectus vel dolor adipiscing tincnt.
More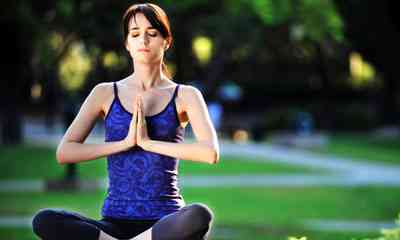
Delecious Trips
Lorem ipsum dolor sit amet consectetur adiing elit. In volutpat luctus eros ac placerat. Quisque erat metus facilisis non feu,aliquam hendrerit quam. Donec ut lectus vel dolor adipiscing tincnt.
MoreLorem ipsum dolor sit
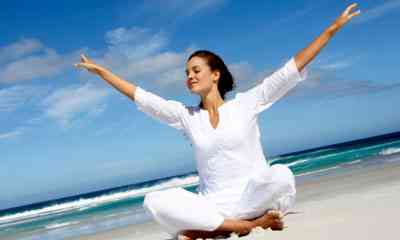
Lorem ipsum dolor sit amet consectetur adiing elit. In volutpat luctus eros ac placerat. Quisque erat metus facilisis non feu,aliquam hendrerit quam. Donec ut lectus vel dolor adipiscing tincnt.
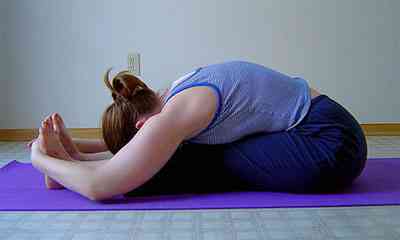
Lorem ipsum dolor sit amet consectetur adiing elit. In volutpat luctus eros ac placerat. Quisque erat metus facilisis non feu,aliquam hendrerit quam. Donec ut lectus vel dolor adipiscing tincnt.
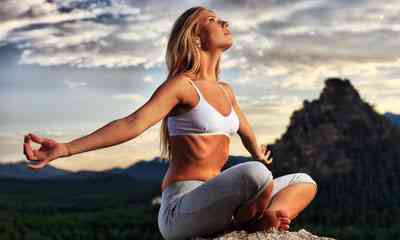
Lorem ipsum dolor sit amet consectetur adiing elit. In volutpat luctus eros ac placerat. Quisque erat metus facilisis non feu,aliquam hendrerit quam. Donec ut lectus vel dolor adipiscing tincnt.